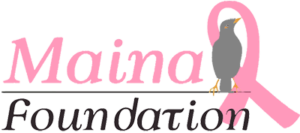
NEWSLETTER
August 2024
Welcome to the Maina Foundation Newsletter!
Artificial Intelligence in Breast Cancer
By
Manju Soni
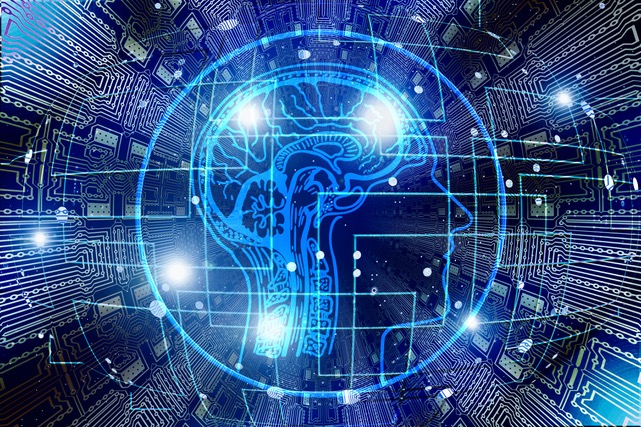
Dear friends
We at Maina Foundation took a short summer break, but we are back and ready to go.
This month, I’ve been pondering all things artificial intelligence (AI). There is so much buzz about AI, and a fair amount of debate on whether it’s useful, a hindrance, or positively dangerous.
What we can all agree on is, the robots are here to stay.
Here are some results from my research that you may find interesting.
What is AI and why is it regarded by some as a threat?
- The defining characteristic of human intelligence is our ability to identify patterns and meaning in those patterns, and to use this prior knowledge to adapt to new conditions. The success of AI is its ability to do the same, and some would say, better.
Some terminology:
- Artificial Intelligence (AI): refers to the broad field of computer science in which machines or algorithms are programmed to simulate human intelligence.
- Machine learning (ML): is a branch of AI in which computers perform defined tasks and apply statistical methods to detect hidden patterns in the data and to improve model performance.
- Deep Learning (DL): is a subfield of ML, where, unlike classic ML, DL does not require humans to find a solution for a task, rather, DL operates by the power of multilayered neural networks, thereby enabling self-discovery of features unknown or unanticipated by humans and eliminating manual human effort for feature extraction.
How is AI already being used in breast cancer:
As expected, with its ability to analyze massive amounts of data faster than you can say “abracadabra,” AI is identifying patterns and risks that even the sharpest human minds might miss.
In addition, technological advancements such as molecular imaging and genomic expression have enabled better cancer characterization, which together with AI have improved our ability to understand cancer.
AI is already being used in various aspects of cancer, and here are a few examples:
- Digital pathology: in the ‘old days’ human pathologists would look at cells on a slide and based on experience and training, differentiate normal cells from cancer cells. Of course, this method was laborious, had significant intra- and inter-observer variability, and sometimes resulted in significant, and tragic, errors.
- Digital pathology transforms histology glass slides into digital images using computerized technology, making it easier to store, and later compare, images, thereby allowing quick disease evaluation and progression.
- AI in digital pathology requires training on good-quality training images, by human experts in the field, who have to manually identify and annotate the regions showing abnormalities or of any pathological significance. With appropriate training, AI can quickly identify cell types accurately and give an insight into histological, morphological, and biologically relevant patterns., with a lower margin of error than manual assessment.
- With regard to breast cancer, AI has increased the odds of identifying metastatic breast cancer in whole slide images of lymph node biopsies.
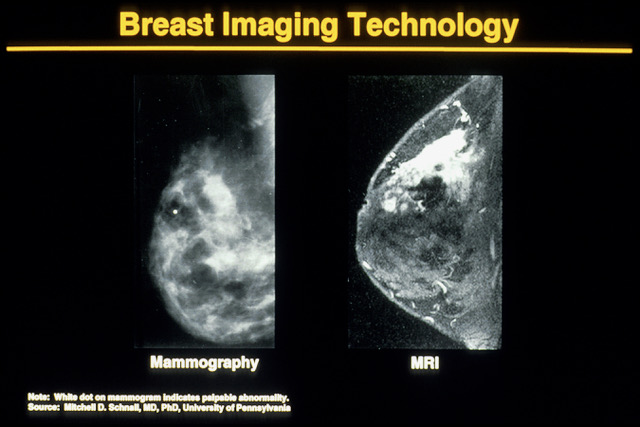
-
Computational radiology
is used in carrying out procedures that were previously carried out by
experts, and it involves computer vision, lesion detection, or
recognition of patterns for lesion detection for classification of
lesions on diagnostic images such as mammograms.
- ML is used to store a large dataset, which is later used to train prediction models and interpret generalizations.
- DL works by establishing a system of artificial neural networks that can read, recognize, and classify images.
- AI in breast cancer screening primarily consists of object detection
(segmentation) and tumor classification as benign or malignant.
Breast masses are common findings in breast cancer patients, thereby making it one of the most important steps in computer-aided diagnosis (CAD). Fuzzy contours are used, which automatically segment breast masses from the mammogram. Breast segmentation is difficult to spot due to irregularities varying from one person to another. Proper segmentation using AI greatly improves the prognosis of the patient.
Calcifications appear as small spots on mammography and are either microcalcification and macrocalcification. At present, CAD systems are capable of detecting microcalcifications.
- Treatment complications: AI has the potential to predict treatment-related toxicity from to radiation and chemotherapy. ML models have been able to predict visits to emergency rooms and hospital admissions due to cancer therapy-related symptoms.
- Survival & disease recurrence: Algorithms for survival prediction have been developed for many cancer types, including breast, prostate and lung cancers. Predicting cancer survival can help tailor treatment strategies. Treatment planning can be reinforced for patients at high risk while interventions with marginal benefit for low-risk patients could be avoided.
In addition, the risk of disease recurrence after curative treatment can be predicted using Al models.
- Artificial intelligence for cancer research: AI has become an important tool in the discovery of potential new drugs in the treatment of cancer.
Clinical trials can also become more efficient with the use of AI. AI has been used to identify patients for clinical trials by incorporating inclusion and exclusion criteria to search for and identify eligible patients. These systems have shown high accuracy while only requiring a fifth of the time used by manual review.
Newer developments with AI in breast cancer:
All guidelines currently in clinical use for breast cancer leverage risk models. These risk models include a patient’s age, hormonal factors, genetics, and mammographic breast density to determine whether supplemental imaging should be considered.
The Tyrer-Cuzick model for example, takes into account various factors in assessing risk, including, age, height and weight, breast density, history and results of breast biopsies, personal history of breast or ovarian cancer, family history of breast or ovarian cancer, history of hormone use, Ashkenazi Jewish heritage, if applicable, age of first menstrual period, age of first giving birth, if applicable, age at menopause, if applicable and age of cancer diagnosis, if applicable.
However, despite decades of effort, the accuracy of risk models used in clinical practice, such as the Tyrer-Cuzick model, remains modest.
More recently, image-based DL models have shown considerable promise.
- AI in breast cancer risk models
This study published in 2021 compared an AI risk model, Mirai, to state-of-the-art clinically adopted Tyrer-Cuzick and other prior deep learning approaches, to assess breast cancer risk from screening mammograms.
Mirai is a mammography-based deep learning model designed to predict risk at multiple timepoints, leverage potentially missing risk factor information, and produce predictions that are consistent across mammography machines.
The results of this study were that Mirai demonstrated improved discriminatory capacity over the more traditional models, including the established Tyrer-Cuzick model.
In addition, Mirai, was tested and found to maintain its performance on datasets from more diverse cohorts without additional training.
In a follow-up study published in 2022, Mirai was validated in a retrospective study. A total of 128,793 mammograms from 62,185 patients were collected across seven sites, of which 3,815 were followed by a cancer diagnosis within 5 years. The seven sites were Massachusetts General Hospital, USA; Novant, USA; Emory, USA; Maccabi-Assuta, Israel; Karolinska, Sweden; Chang Gung Memorial Hospital, Taiwan; and Barretos, Brazil.
The results show, Mirai, a mammography-based risk model, maintained its accuracy across globally diverse test sets from seven hospitals across five countries.
What are the downsides to AI in oncology
A big caveat to the utility of these models is the old computer science concept that flawed, biased or poor quality input produces a result or output of similar quality.
AI is based on data collected from specific populations, and has been shown to have an intrinsic bias against underrepresented persons. Therefore, a disparity is sure to arise when it comes to apply the AI to people belonging to different race and/ or socioeconomic conditions.
Cancer is also a particular disease that has manifestations and incidences that vary across different races. Because people's risk factors and predispositions differ, AI algorithms operate differently in different populations. To bring an image-based risk model to the clinic, we not only need to further improve its accuracy but must also validate its performance across diverse populations and clinical settings, because multiple studies have demonstrated that transferability of AI tools should not be taken for granted.
Another limitation is AI’s “black box” mechanism. For AI machines to be truly accepted, people must be able to independently replicate and produce the machine like any other scientific finding.
However, in spite of good intent, the lack of standardization across health care systems and global communities often limits widespread exchange of health data and information.
This, in turn, limits the reproducibility of AI models and perpetuates health care disparities.
Ethical concerns are also very important. Using AI methods need to consider data confidentiality, privacy violation, the autonomy of patients, consent, etc.
In Conclusion
According to the World Health Organization, breast cancer is the commonest cancer worldwide and the fifth most common cause of cancer deaths.
The incorporation of AI into different screening and treatment methods has made it easier to diagnose and treat cancer early.
However, the use of AI has its limitations as well as ethical concerns around patient privacy.
As this technology continues to advance, its role in improving patient outcomes and the overall effectiveness of breast cancer screening will offer hope for a future where breast cancer can be detected and treated more effectively.
To our Maina Foundation supporters, thank you for reading, and your valuable contributions.
Please help us save more lives by forwarding the newsletter. Thank you!
Manju Soni (she/her) (pen name: M. J. Soni) is a former eye surgeon turned author. She is the author of Defying Apartheid, her debut nonfiction book that captures her experiences of being a young activist against apartheid. Her short fiction and essays has appeared in Ellery Queen Mystery Magazine, Akashic Books, Apeiron Review and The Establishment. She’s a member of Crime Writers of Color and Sisters in Crime (National and Connecticut).
https://manjusoni.com
Stay Tuned!
We have some exciting fundraising possibilities coming up in September for October Breast Cancer Prevention Month.
We thank you for your continued support!
For more information on Maina Foundation, and to help its mission, go to https://mainafoundation.org or contact us at 860-434-3985 or info@mainafoundation.org
SAVE A LIFE - DONATE NOW
Donations can also be mailed to:
8 Peppermint Ridge, Old Lyme, CT 06371, USA